Study: When allocating scarce resources with AI, randomization can improve fairness
Organizations are increasingly utilizing machine-learning models to allocate scarce resources or opportunities. For instance, such models can help companies screen resumes to choose job interview candidates or aid hospitals in ranking kidney transplant patients based on their likelihood of survival.When deploying a model, users typically strive to ensure its predictions are fair by reducing bias. This often involves techniques like adjusting the features a model uses to make decisions or calibrating the scores it generates.
However, researchers from MIT and Northeastern University argue that these fairness methods are not sufficient to address structural injustices and inherent uncertainties. In a new paper, they show how randomizing a model’s decisions in a structured way can improve fairness in certain situations.
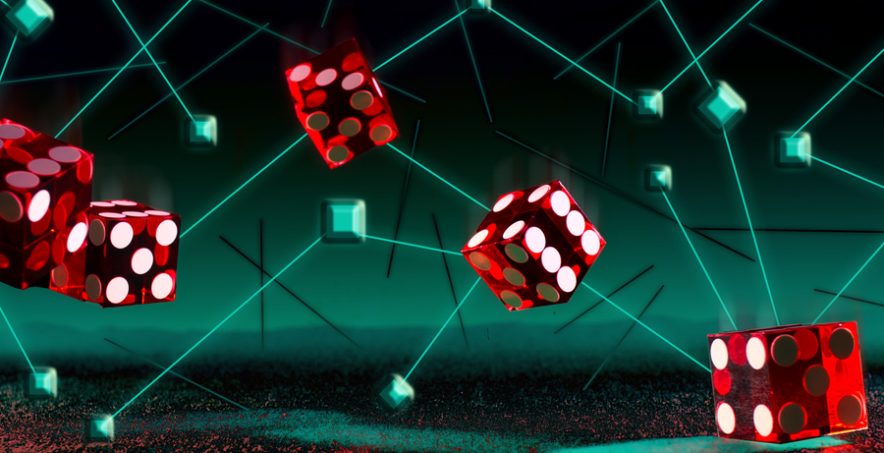