MIT scientist is using AI to develop biomarkers for Rett Syndrome
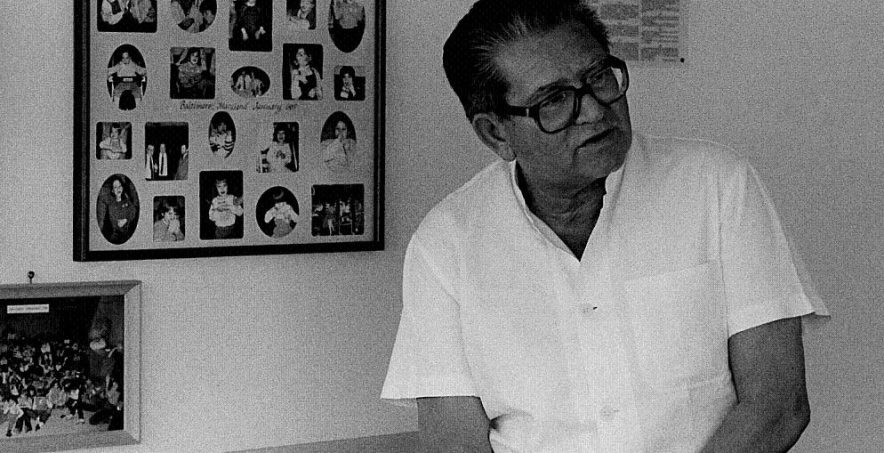
With an award of $1.1 million, Emerald Innovations, a spinout by MIT professor Dina Katabi, is working with the Rett Syndrome Research Trust to use a contactless AI device to remotely measure the symptoms of a rare neurological disorder.
In a waiting room in postwar 1960s Austria, two girls sat in their mothers’ laps making repetitive hand-washing motions as their mothers attempted to gently restrain them. The pair caught the sharp eyes of Viennese pediatrician Dr. Andreas Rett, who would later observe that the two unrelated girls shared distinctly similar clinical and developmental histories: typical initial development milestones in their first nine months, followed by a loss of previously acquired skills. It wasn’t until 1982 that the neurological disorder achieved enough recognition to be named Rett Syndrome. Forty years later, Rett is now studied in hundreds of academic labs across the globe and more recently has drawn the attention of the biopharmaceutical industry.
With several drugs advancing toward clinical testing, the ability to objectively measure Rett clinical symptoms becomes critical. Yet, like many other neurological disorders, Rett lacks quantifiable objective measures. As a result, new drugs and therapies are typically evaluated using semi-subjective questionnaires. With this recent award, Dina Katabi, Thuan (1990) and Nicole Pham Professor in the Department of Electrical Engineering and Computer Science (EECS) at MIT and co-founder of Emerald Innovations, is working with the Rett Syndrome Research Trust (RSRT) on a clinical study designed to monitor Rett symptoms and develop objective markers of the disease.
The study will leverage the Emerald biosensor, a device that uses machine learning to analyze the wireless signals that it emits into the environment (like a bat using echolocation) to measure critical biomarkers 24/7, without the need for patients to wear sensors on their bodies. The device has already been used in other studies to monitor various diseases like Parkinson’s, Alzheimer’s, Crohn’s, COVID-19, and Atopic Dermatitis (eczema).
RSRT, the leading non-profit that aims to find a cure for Rett Syndrome, hopes to make progress in monitoring responses to drug and genetic treatment for Rett using Emerald’s ability to passively monitor physiological signals like breathing, sleep, and movement.
“Neurological disorders typically lack objective and sensitive measures, which negatively impact the ability to understand such diseases and develop drugs for them,” says Katabi, an affiliate of Jameel Clinic for Machine Learning in Health (Jameel Clinic) and the Computer Science and Artificial Intelligence Laboratory (CSAIL). “We are working to change the status quo and enable objective, sensitive AI-based disease markers.”
Individuals with Rett exhibit a variety of symptoms including but not limited to: loss of purposeful hand use, loss of speech and coordinated movement, sleep issues, and breathing irregularities. An earlier pilot study demonstrated that the Emerald biosensor was able to capture significant abnormalities in patients’ sleep and breathing over a four-week period. The current study, which has a $1.1 million budget, will build and expand on the pilot study by not only measuring sleep and breathing, but also characterizing movement.
“My colleagues and I are delighted to be working with Dina and the cutting-edge technology that she has developed. The ability to objectively measure symptoms that are clinically relevant is critical.” Monica Coenraads, CEO of RSRT, says. “We are optimistic that Emerald can help us achieve that and we are grateful to RSRT’ trustee, Alba Tull, for supporting this project with a $500,000 lead gift.”
In recent years, funding for AI and digital technologies in health has reached record highs. Katabi emphasized the need for a data-driven approach to healthcare, noting the importance of ensuring that research is able to transition from the academic domain to the translational phase, i.e. applying the research to clinical practice. “As a professor, I encourage my students to consider research at the intersection of machine learning and biomedicine and, whenever possible, work to see their results improve the lives of patients.”